How Much More Data Will AI Generate? 10x, 100x, 1000x?
Artificial intelligence and GenAI may give new meaning to 'big data'
Welcome to the Cloud Database Report. I’m John Foley, a long-time tech journalist, including 18 years at InformationWeek, who worked in strategic comms at Oracle, IBM, and MongoDB. I’m now a VP with Method Communications.
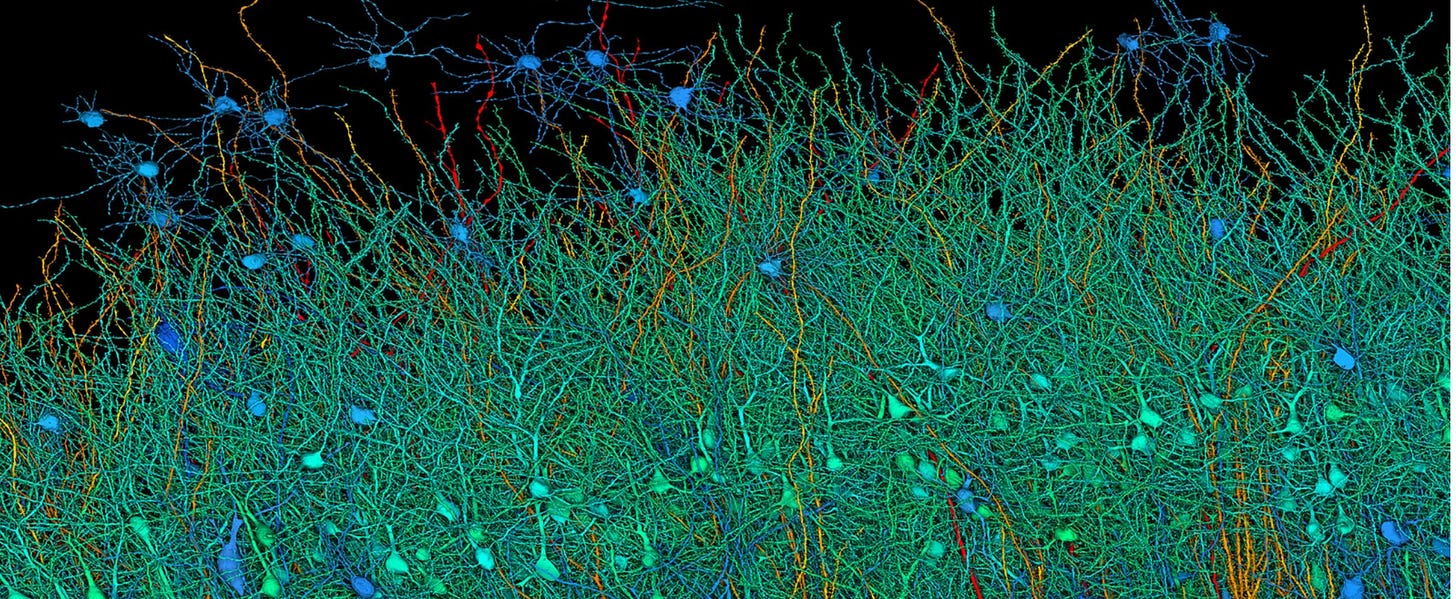
Is data volume, driven by AI, about to hit the proverbial “hockey stick” with a sharp upward turn?
Much of the talk recently has been about LLMs’ insatiable appetite for Web data. That’s not what this blog post is about.
I’m curious about how much new data will be generated by AI systems and applications, and I believe it’s going to be a big multiple. Maybe 10, 100, or even 1,000 times more data within the next few years. Today’s petabytes, could be tomorrow’s AI-fueled exabytes.
These are scratchpad calculations, so let me explain. It’s hard to find forecasts of AI-related data growth, but there’s plenty of anecdotal evidence. Consider:
Data is already growing fast. IDC, in its Global DataSphere Forecast, has estimated that data volume would double from 2023 to 2027. That’s 2x, or 100%, growth in four years. Yet, I take that as a conservative estimate that may have preceded the release of ChatGPT in late 2022. I’m betting that AI will have a multiplier effect on these and other “pre-AI” metrics. Which is to say, IDC’s estimate may be on the light side when you factor in GenAI.
Data center storage is poised for a “strong rebound” after a decline in 2023, according to Omdia. AI data is part of the reason. The storage rebound will be driven by “digital transformation projects within enterprises, data volume growth, AI advances pulling storage in their wake, and a general need for storage modernization.” That’s courtesy of Block & Files, citing Omdia’s forecast.
Interest in vector databases, which are used to manage AI/vector data, is booming. They’re now the most popular type of databases tracked by DB Engines, according to Alex Woodie at Datanami. The DB Engines website lists 15 vector databases and that jumps to 26 if you include databases with a secondary model. The most comprehensive list I’ve seen was compiled by Christoph Bussler, who has identified nearly 150 vector databases. Why so many? Because more and more data is being vectorized.
Capital spending is up across the board as hyperscalers build out capacity for AI workloads. Bob Evans, my three-time former colleague and Editor of Cloud Wars, recently calculated the total cap investment by the big four hyperscalers — Microsoft, AWS, Google, and Oracle — to be at an eye-popping $200 billion+ annual run rate. Much of that investment will go to infrastructure for model training and AI workloads, but I would bet that scaling for AI-generated data is baked into the planning. If you need a proof point, look no further than the rebound in storage systems mentioned above.
If you have to see it to believe it, check out the impressive new AI data center (pictured below) that Oracle built near Salt Lake City. The facility houses 16,000 GPUs and is still growing. Oracle describes it as one giant GPU cluster. The company recently provided a walk-through of the data center.
AI gives new meaning to ‘big data’
Let’s say that you’re not yet convinced by my premise — that AI is poised to create 10, 100, or 1,000 times more data (text, GenAI-created images/video/audio, vector embeddings, graph, time series, IoT, and so on).
How about this: Google scientists and Harvard neuroscientists recently created a 3D map of six layers of the human brain that required 1.4 petabytes to encode. “This is the largest dataset ever made of human brain structure at this resolution,” the researchers wrote in a blog post.
They did this with AI-based image processing and analysis. And notably, the sample was small — just one millionth of the human brain. Just imagine the datasets involved as this kind of AI-enabled analysis is brought to all kinds of research and problem solving. Many, many petabytes.
This important work, known as connectomics, is being applied to the study of neurological diseases such as Alzheimers. You can read a more detailed article about it here.
My old rule: 1 million times more data every 25 years
Long-time readers of the Cloud Database Report will recall my rule of thumb — that, based on my experience in enterprise tech, we’ve witnessed data growth of about 1 million times over the past 25 years. In a nutshell, large-scale data estates have grown from terabytes to petabytes, and more recently exabytes, over that time span. (In the article below, I also explain how this aligns with Moore’s Law.)
So, with this trend line in mind, it’s not a stretch to think that AI will add fuel to the data boom already underway. The only questions are: How much more data? And how fast?
Your guess is as good as mine.
What if I’m wrong. Is it possible that AI, due to its intelligence, automation, and efficiency, could actually contain data growth? I don’t think so. GenAI, by definition, is about generation. And that means more data.
In the weeks ahead, I will be exploring these questions in conversations with Oracle, Salesforce, Yugabyte, and Cockroach Labs. I welcome your thoughts. Please feel free to leave a comment here or connect with me on LinkedIn.